Boosting: Foundations and Algorithms
by Robert E. Schapire, Yoav Freund
Publisher: The MIT Press 2014
ISBN-13: 9780262310413
Number of pages: 544
Description:
Boosting is an approach to machine learning based on the idea of creating a highly accurate predictor by combining many weak and inaccurate 'rules of thumb'. A remarkably rich theory has evolved around boosting, with connections to a range of topics, including statistics, game theory, convex optimization, and information geometry.
Download or read it online for free here:
Read online
(online html)
Similar books
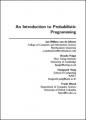
by Jan-Willem van de Meent, et al. - arXiv.org
This text is designed to be a graduate-level introduction to probabilistic programming. It provides a thorough background for anyone wishing to use a probabilistic programming system, and introduces the techniques needed to build these systems.
(4869 views)
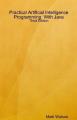
by Mark Watson - Lulu.com
The book uses the author's libraries and the best of open source software to introduce AI (Artificial Intelligence) technologies like neural networks, genetic algorithms, expert systems, machine learning, and NLP (natural language processing).
(24665 views)
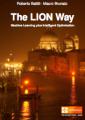
by Roberto Battiti, Mauro Brunato - Lionsolver, Inc.
Learning and Intelligent Optimization (LION) is the combination of learning from data and optimization applied to solve complex problems. This book is about increasing the automation level and connecting data directly to decisions and actions.
(35717 views)
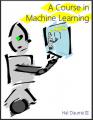
by Hal Daumé III - ciml.info
Tis is a set of introductory materials that covers most major aspects of modern machine learning (supervised and unsupervised learning, large margin methods, probabilistic modeling, etc.). It's focus is on broad applications with a rigorous backbone.
(21697 views)