A Brief Introduction to Machine Learning for Engineers
by Osvaldo Simeone
Publisher: arXiv.org 2017
Number of pages: 237
Description:
This monograph aims at providing an introduction to key concepts, algorithms, and theoretical results in machine learning. The treatment concentrates on probabilistic models for supervised and unsupervised learning problems. It introduces fundamental concepts and algorithms by building on first principles, while also exposing the reader to more advanced topics with extensive pointers to the literature, within a unified notation and mathematical framework.
Download or read it online for free here:
Download link
(2.1MB, PDF)
Similar books
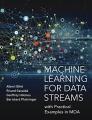
by Albert Bifet, et al. - The MIT Press
This book presents algorithms and techniques used in data stream mining and real-time analytics. Taking a hands-on approach, the book demonstrates the techniques using MOA, allowing readers to try out the techniques after reading the explanations.
(6442 views)
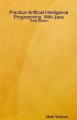
by Mark Watson - Lulu.com
The book uses the author's libraries and the best of open source software to introduce AI (Artificial Intelligence) technologies like neural networks, genetic algorithms, expert systems, machine learning, and NLP (natural language processing).
(24574 views)
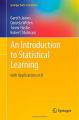
by G. James, D. Witten, T. Hastie, R. Tibshirani - Springer
This book provides an introduction to statistical learning methods. It contains a number of R labs with detailed explanations on how to implement the various methods in real life settings and it is a valuable resource for a practicing data scientist.
(9856 views)
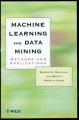
by Aaron Hertzmann - University of Toronto
Contents: Introduction to Machine Learning; Linear Regression; Nonlinear Regression; Quadratics; Basic Probability Theory; Probability Density Functions; Estimation; Classification; Gradient Descent; Cross Validation; Bayesian Methods; and more.
(10008 views)