Reinforcement Learning
by C. Weber, M. Elshaw, N. M. Mayer
Publisher: InTech 2008
ISBN-13: 9783902613141
Number of pages: 424
Description:
The first 11 chapters of this book describe and extend the scope of reinforcement learning. The remaining 11 chapters show that there is already wide usage in numerous fields. Reinforcement learning can tackle control tasks that are too complex for traditional, hand-designed, non-learning controllers. As learning computers can deal with technical complexities, the tasks of human operators remain to specify goals on increasingly higher levels.
Download or read it online for free here:
Download link
(12MB, PDF)
Similar books
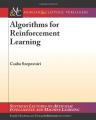
by Csaba Szepesvari - Morgan and Claypool Publishers
We focus on those algorithms of reinforcement learning that build on the theory of dynamic programming. We give a comprehensive catalog of learning problems, describe the core ideas, followed by the discussion of their properties and limitations.
(7794 views)
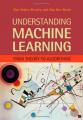
by Shai Shalev-Shwartz, Shai Ben-David - Cambridge University Press
This book introduces machine learning and the algorithmic paradigms it offers. It provides a theoretical account of the fundamentals underlying machine learning and mathematical derivations that transform these principles into practical algorithms.
(10219 views)
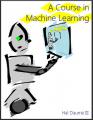
by Hal Daumé III - ciml.info
Tis is a set of introductory materials that covers most major aspects of modern machine learning (supervised and unsupervised learning, large margin methods, probabilistic modeling, etc.). It's focus is on broad applications with a rigorous backbone.
(21643 views)
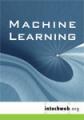
by Abdelhamid Mellouk, Abdennacer Chebira - InTech
Neural machine learning approaches, Hamiltonian neural networks, similarity discriminant analysis, machine learning methods for spoken dialogue simulation and optimization, linear subspace learning for facial expression analysis, and more.
(16280 views)